DeepTCR
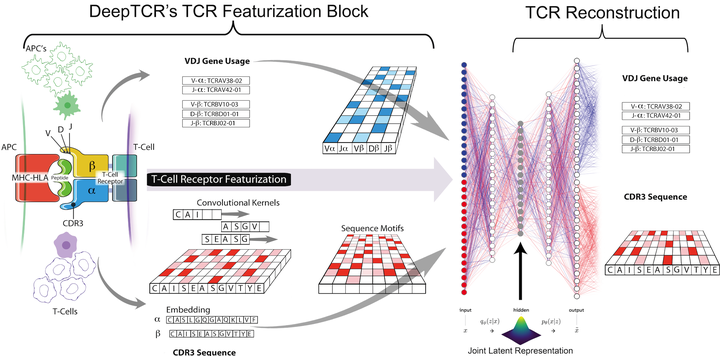
The Story
The idea for DeepTCR has very interesting origins. When I first became involved in the field of cancer immunology, I was interested in studying T-cell receptor (TCR) repertoire and one of my first project was developing an algorithm called ImmunoMap. ImmunoMap was an algorithm to capture the structural diversity of T-cell repertoires; however, one of the shortcomings of the method was that it required quite a bit of manual parameter tuning. This made it quite difficult to use quickly and easily.
In 2017, while I was at the AACR Annual Meeting to give an oral presentation on ImmunoMap, I attended another talk given by Google where they were presenting on applications of deep learning within the medical sciences. As I listened to the talk, it hit me that deep learning was the methodological approach that made sense for analyzing TCR repertoire data. During that talk, I purchased THE textbook on deep learning, and decided I was going to learn how to apply deep learning to the analysis of TCR repertoire data. When I came back to Hopkins, I proposed the idea to my mentors and thus, this is how I dived into the world of deep learning and led to the creation of DeepTCR.
After 4 years from the time I conceived of DeepTCR, we finally had our work published in Nature Communications in February of 2021. Feel free to check out the manuscript here or at the publisher’s website here
Abstract
Deep learning algorithms have been utilized to achieve enhanced performance in pattern-recognition tasks. The ability to learn complex patterns in data has tremendous implications in immunogenomics. T-cell receptor (TCR) sequencing assesses the diversity of the adaptive immune system and allows for modeling its sequence determinants of antigenicity. We present DeepTCR, a suite of unsupervised and supervised deep learning methods able to model highly complex TCR sequencing data by learning a joint representation of a TCR by its CDR3 sequences and V/D/J gene usage. We demonstrate the utility of deep learning to provide an improved ‘featurization’ of the TCR across multiple human and murine datasets, including improved classification of antigen-specific TCRs and extraction of antigen-specific TCRs from noisy single-cell RNA-Seq and T-cell culture-based assays. Our results highlight the flexibility and capacity for deep neural networks to extract meaningful information from complex immunogenomic data for both descriptive and predictive purposes.
Reflection
This project is a particularly special one for me because of the ownership I had over it since its conception. In general, I have been lucky to have mentors throughout my graduate training who have given me complete autonomy to work on my own ideas; often at the risk of failing given the nature of the projects I was pursuing. In fact, when I first proposed this particular idea of using deep learning in TCR-Seq, it was not met with the enthusiasm I thought I would get. I had to really push on this idea on my own until I had preliminary results that suggested this would be a worthwhile endeavor. Throughout the process, I learned to trust my intuition and vision for this project, which became difficult as this manuscript actually went into review at 3 separate journals with 10 different reviewers. With each review/rejection, I had to pick myself up, re-work the manuscript/analyses, and try again. This whole process took over 2 years from the time of the very first submission to its eventual acceptance. By the time it was eventually accepted, I cannot explain the relief and satisfaction of seeing this project go from conception to actualization. That being said, if your work uses T-cell receptor repertoire sequencing, give DeepTCR a look! And if you have any questions, I’m more than happy to help get you started with it!