DeepAPL
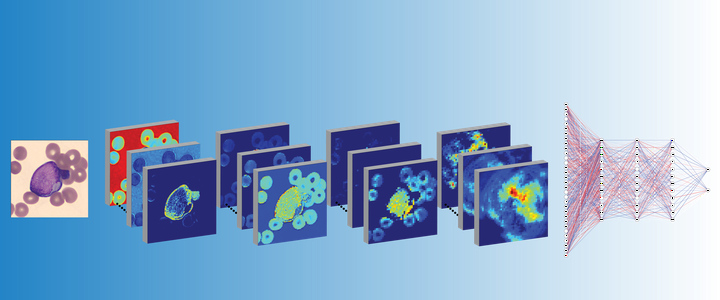
Background
It was the summer of 2017 when I was attending our annual Bloomberg~Kimmel Institute for Immunotherapy meeting where my paths crossed with Eugene Shenderov, at the time, a recently finishing oncology fellow at Hopkins. We hit it off at the poster session and after the day was over, we stood outside in the parking lot and talked for at least an hour or more about cool scientific opportunities. He realized I had an interest in machine learning and proposed a project he had been thinking about to leverage machine learning/AI to diagnose Acute Promyelocytic Leukemia (APL). He presented the problem as this rare but quickly fatal subtype of Acute Myeloid Leukemia (AML) that current clinicians had a difficult time quickly diagnosing as the gold standard test could take days to result while APL, a true oncologic emergency, could prove fatal within hours. I thought the idea was fascinating and had recently been acclimating myself to deep learning techniques, and thought, this could be an excellent project to continue to refine those skills. We then began working together along with a team of medical students and experts in leukemia at Johns Hopkins to develop a deep learning algorithm that could quickly and accurately diagnose APL from peripheral smear at the time of clinical presentation. Almost 4 years later, we finally published a manuscript in npj Precision Oncology describing our algorithm and demonstrating the ability for our deep learning model to identify patients with APL in a independent prospective cohort of patients. We hope to expand this soon to a larger multi-institutional study to move this towards a helpful physician-aid in the diagnosis and management of acute leukemia.
Abstract
Acute Promyelocytic Leukemia (APL) is a subtype of Acute Myeloid Leukemia (AML), classified by a translocation between chromosomes 15 and 17 [t(15;17)], that is considered a true oncologic emergency though appropriate therapy is considered curative. Therapy is often initiated on clinical suspicion, informed by both clinical presentation as well as direct visualization of the peripheral smear. We hypothesized that genomic imprinting of morphologic features learned by deep learning pattern recognition would have greater discriminatory power and consistency compared to humans, thereby facilitating identification of t(15;17) positive APL. By applying both cell-level and patient-level classification linked to t(15;17) PML/RARA ground-truth, we demonstrate that deep learning is capable of distinguishing APL in both a discovery and prospective independent cohort of patients. Furthermore, we extract learned information from the trained network to identify previously undescribed morphological features of APL. The deep learning method we describe herein potentially allows a rapid, explainable, and accurate physician-aid for diagnosing APL at the time of presentation in any resource-poor or -rich medical setting given the universally available peripheral smear.